EkkoSense data center management guides
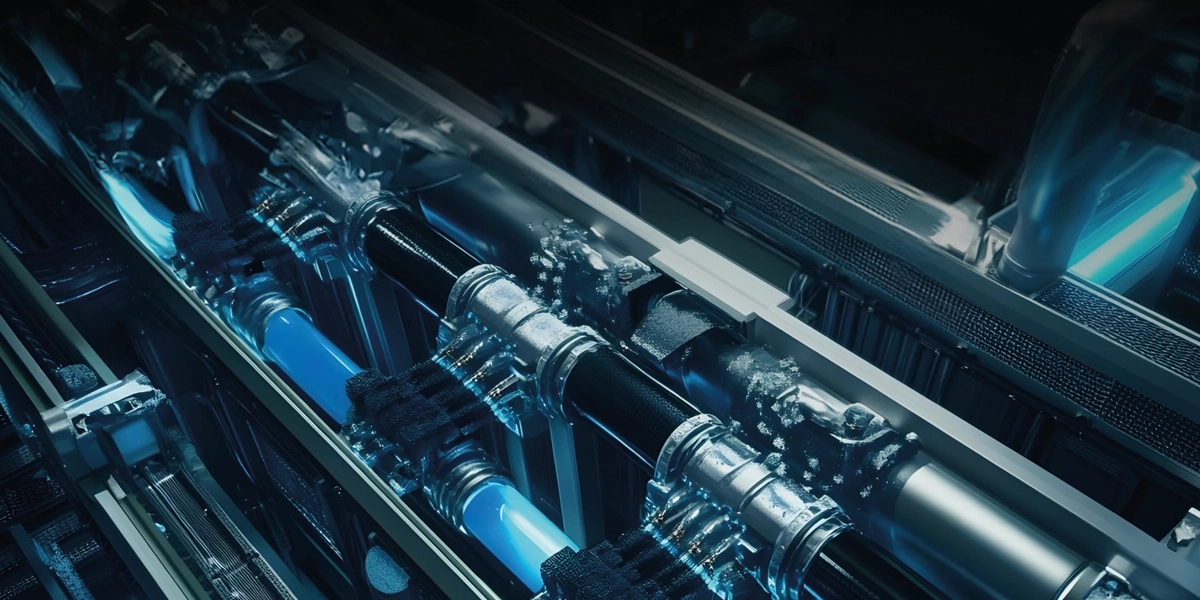
AI compute growth is leading to some very real data center engineering challenges
Data center management guide.
We’re quickly moving towards a world where almost all organizations will have their own AI applications – some deployed tactically, while others will be used more strategically to remodel core processes and unlock new levels of efficiency.
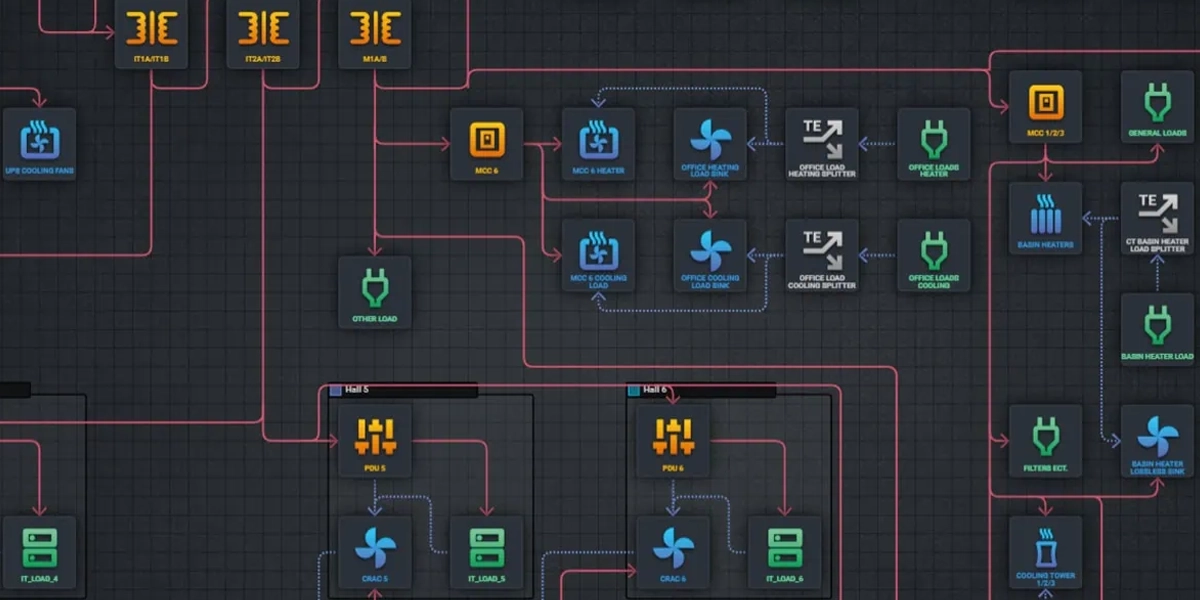
How data center digital twin simulations are set to derisk the transition to AI compute
Data center management guide.
With AI remodeling today’s data centers, IT teams must focus on derisking its impact. A new generation of data center digital twin and data center simulation will be critical to their success.
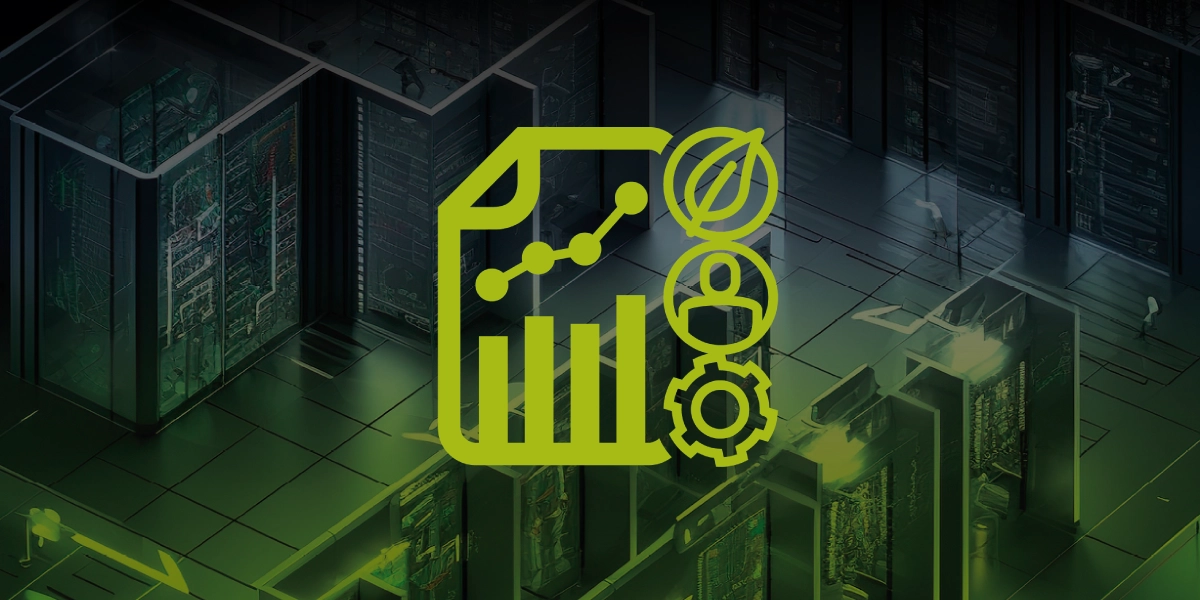
ESG and Climate Accountability Reporting for U.S. Data Centers
Data center management guide.
Environmental, Social and Governance (ESG) issues are rapidly evolving from a largely corporate and social responsibility issue into a core compliance challenge for U.S. data center operators.
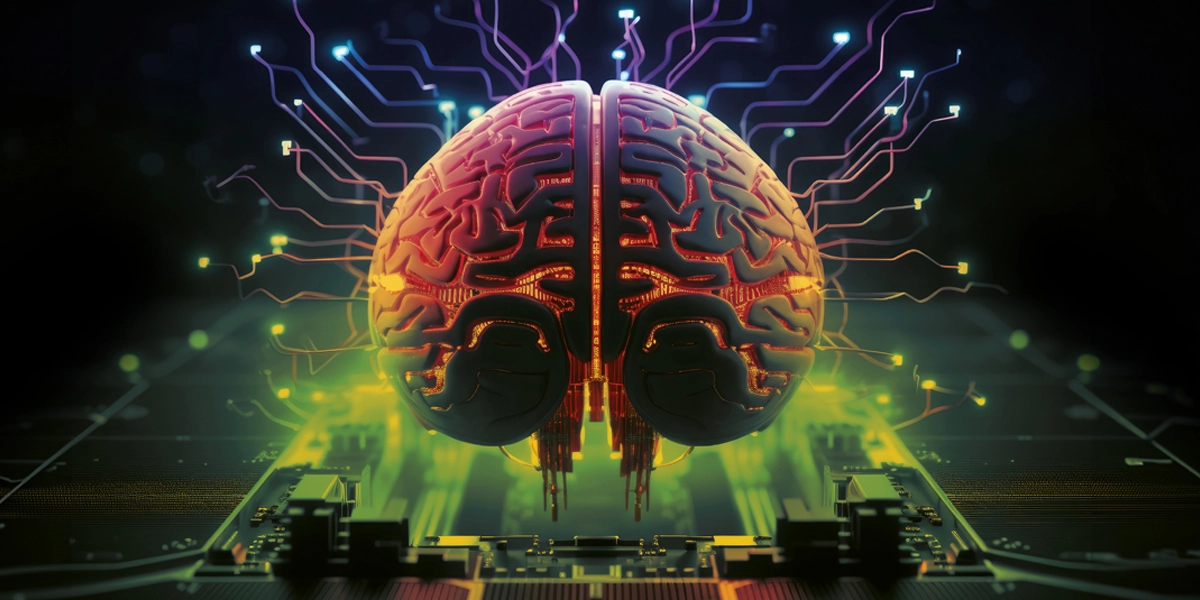
Data center AI – Accelerate your time to AI compute benefits
Data center management guide.
Gen AI deployments: how ready are we really? Navigating the AI gold rush in data center management.
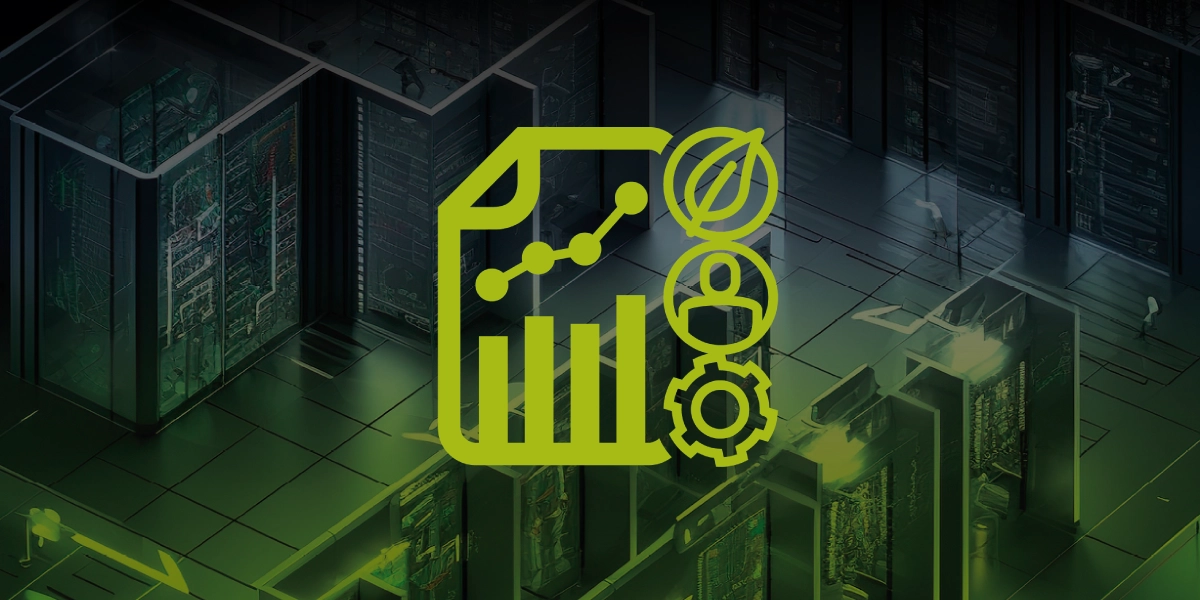
Are you ready for data center ESG Reporting?
Data center management guide.
ESG set to evolve from being a largely Corporate & Social Responsibility issue to become a core compliance challenge for data centre operators.
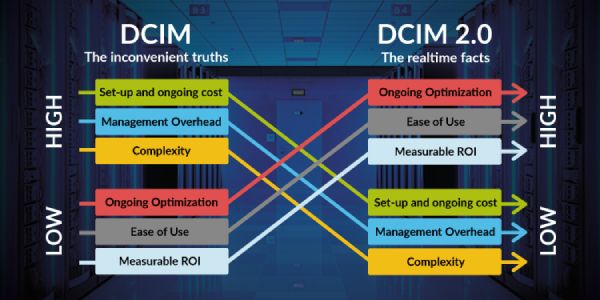
DCIM still matters – but it has to deliver and pay for itself this time.
Data center management guide.
Generally, DCIM has failed to deliver on the promises made – indeed in many cases it has turned out to be little more than a stronger Building Management System or an over-priced Asset Register. This has left many data center operations teams – and their CIOs and CFOs – quite rightly asking questions such as ‘what has DCIM ever done for us?’ and ‘where’s the return on our DCIM investment?’. Unfortunately, too many organizations with major DCIM projects in play find these questions just too difficult to answer.
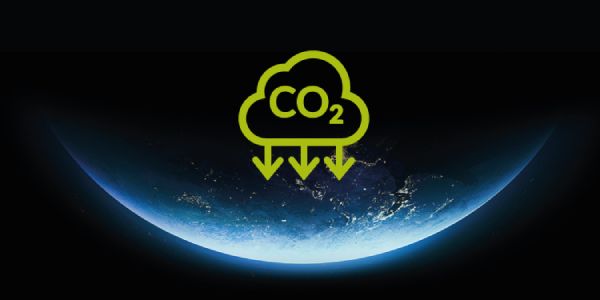
Cutting your data center energy usage by a third.
Data center management guide.
At a time when organizations worldwide are committing to ambitious net zero carbon reduction targets, new research from data center performance optimization specialist EkkoSense suggests that many operations are missing out on proven ways to reduce their cooling energy consumption.
Connect with EkkoNet Global Partners
EkkoSense data center partners. Internationally recognized consulting and knowledge base, universally trusted delivery solutions, world class regional support.
Talk to an EkkoSense Expert
Get in touch with questions, sales enquiries or to arrange your free demonstration.